Building on Best Practices to Limit Risk in AI Systems
By ImageSource Team on May 24, 2024
AI is Already in Use Within Your Organization (Whether Sanctioned or Not)
Microsoft and LinkedIn recently published their 2024 Work Trend Index Annual Report titled AI at Work is Here. Now Comes the Hard Part. It found that 75% of knowledge workers use AI at work, with 78% bringing their own tools (using open AI). There’s a silver lining for leaders who worry about productivity and how to measure it: 90% of users categorized as “power users” state that AI “makes their overwhelming workload more manageable and their work more enjoyable.”
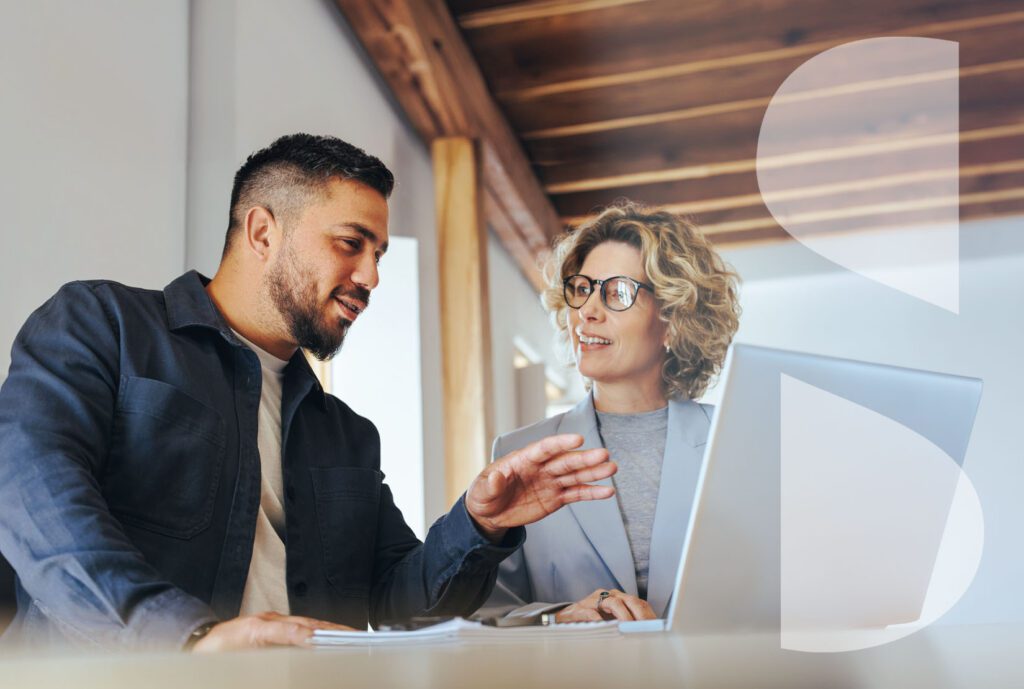
When it comes to modern AI applications, we’re still contending with the fear, uncertainty, and doubt around AI gone wrong. The examples are, at times, either comical or harrowing. All serve as a cautionary tale for not adequately addressing the risks associated with AI.
AI systems are human-engineered, machine-based systems that use targeted prompts to generate outputs, including predictions, recommendations, or creative works. They are designed to operate with varying degrees of autonomy.
Because AI applications are trained on human-made source material, they are influenced by social dynamics and human biases. These affect the content and reliability of AI output, running the risks of machine-generated content presented as fact, false attributions, social discrimination, or business recommendations that result in adverse economic outcomes.
The steps required to ensure reliable, trustworthy, and accurate AI output are relatively the same whether you are using a third-party vendor (cloud or otherwise) or your own trained models—with one major exception. If you rely on third-party models, you’ll never have access to examine the training data and understand how it maps to your own data. If you elect to establish your own containerized AI system, you have control over the training data and detailed knowledge of it.
Utilize Existing Frameworks for Planning
Organizations looking to manage risk in AI systems can reference many frameworks for guidance. For instance, the National Institute of Standards and Technology (NIST) has published an Artificial Intelligence Risk Management Framework (AI RMF) for traditional AI and generative AI. This framework breaks risks into categories based on the duration of negative outcomes, probability of occurrence, whether risks are systemic or localized, and severity of impact. The negative outcomes of AI may be harmful to people and communities, organizations, or even economic systems.
This framework establishes a hierarchy to help users assess the trustworthiness of various AI models and systems. It lays out a four-point core strategy for controlling risks:
- Govern: Create a culture of risk management in your organization
- Map: Recognize the context in your data and identify the risks inherently related
- Measure: Track instances of risk and assess their impact
- Manage: Prioritize risk response and take actions to limit occurrence
Curate Your Training Data Carefully to Guard Against Bias
To limit the impact of bias on your AI system, build on data grounded in fact instead of human creativity or subjectivity. Assemble and evaluate source data carefully to ensure that you are training and testing your AI projects on ground truth data.
Ground truth data is defined as “information that is known to be real or true, provided by direct observation and measurement (i.e., empirical evidence) as opposed to information provided by inference.” It is considered authentic, accurate information and used as a reference or benchmark in machine learning, remote sensing, and data analysis.
Begin by defining, collecting, and normalizing a test data set representative of the data you plan to use in production. A data scientist or trusted project team can help you curate quality data, preprocess and normalize it, and test it during training. Once it is annotated with the actual outcomes or results from natural operations, it becomes ground truth data. This data is then used to compare the output of AI models and calculate error rates, including false positives and negatives.
Solidify a Test Strategy
Once a framework is adopted, your organization should define a testing strategy. The extent of control available to you depends on the level of access to an AI model.
Your organization can adopt established best practices to ensure your AI system meets your requirements. These generally include:
- Data quality assurance: Ensure that the data used for training and testing is accurate, relevant, and representative of the problem domain
- Bias evaluation: Use techniques like demographic parity, equalized odds, and disparate impact analysis to assess the AI for bias related to gender, race, age, and other sensitive attributes.
- Performance metrics: Define appropriate evaluation metrics based on the specific task and objectives of the AI model
- Collaboration and feedback: Foster communication between data scientists, domain experts, and other stakeholders during testing; solicit input from diverse perspectives to improve the effectiveness of the testing strategy.
This framework will help you navigate your way to a solid, defensible, and comprehensive testing strategy that verifies the performance of the AI models and establish a plan for implementing and repeating testing to ensure ongoing reliability.
Conclusion
When it comes to implementing an AI system, deciding where to start can be overwhelming. It’s easy to zero in on AI technology offerings while overlooking critical considerations around implementing AI safely, reliably, and responsibly. Ultimately, having a solid AI framework that defines the acceptable use of AI within your organization, how to test it, and how to operationalize it are the critical factors for limiting risk and achieving successful outcomes.